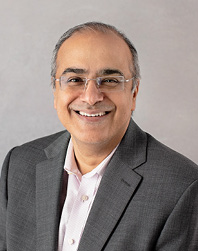
There’s no turning back when it comes to digital transformation in the tax space. But are you prepared to deal with it head on? To get a professional perspective, we turned to an expert who’s been navigating this digital sea change from its beginnings. Satnam Singh, chief product officer for corporate tax and trade at Thomson Reuters, has twenty-one years of experience across three related domains: digital transformation, data analytics and artificial intelligence (AI), and product management. Educated in the computer science department at MIT, Singh started this journey at Akamai Technologies, a leading content distribution network. As part of the company’s network team, Singh wrote advanced algorithms for content routing and optimization. He then joined Keynote Systems, one of the first software-as-a-service (SaaS) companies to deliver performance monitoring, optimization, and customer experience measurement tools. Since then Singh has led several teams in various combinations of these specialty areas.
Michael Levin-Epstein, Tax Executive’s senior editor, conducted this interview with Singh in early March.
Michael Levin-Epstein: Can you describe your role at Thomson Reuters?
Satnam Singh: My role at Thomson Reuters covers three areas. The first one is as the head of product for the corporate tax and trade division, which covers direct tax, indirect tax, global trade, trust, global compliance reporting, and our platform services. In addition, I also lead the team for tax and trade content; our customers need accurate, up-to-date information regarding regulatory compliance from our software, and this is the team that looks at all the regulatory changes that are happening, whether [they are] for tax or global trade, and incorporates them into our software. And then the third hat that I wear is as the leader of the API [application programing interface] and cloud strategy across all applications for TR.
Levin-Epstein: What has happened in the tax world for digital transformation to be such a dynamic phenomenon in the last five years or so?
Singh: I think there are a few systemic changes that have happened, Michael. At a macro level, if you will, there are two. One is the one that’s being introduced by the governments looking to make tax collection and doing insights on the tax collection easier for them. The second is the corporations themselves looking to drive more efficiency and insights into processes related to tax compliance. Now, let’s peel that onion a little bit more. If you look at it from a government perspective, around the world governments are looking, more so on an ongoing basis, to indirect tax as a way to balance their budgets from the revenues they’ve lost from direct tax. However, with indirect tax the volume of the data is much larger and the complexity much higher in terms of various entities involved, and therefore governments are trying to digitize the related operations. Governments are looking to make it easier for businesses to file indirect taxes, get more insights, and discourage tax fraud.
For example, if you take a look at the European Commission’s 2020 legislative proposal, it was all about enhancing data collection about e-commerce transactions. The payment service providers, like banks and e-wallets, now transfer information to EU member states about cross-border payments. Then the idea is to centralize that information into a European database. So that’s one example of the shift to digital for tax-related operations. The second is the governments themselves who are going through a lot of changes in terms of the digitization of the processes and workflow related to tax filing and auditing. That was the first of the two macro changes I referred to earlier.
The second macro change is coming from corporations, organizations, who are looking at their workflows related to tax and trade, with the goal to shift it more towards automation and away from manual operations. This is where they’re trying to kind of shift their workflows and processes to utilize technologies like analytics, robotic process automation, workflow automation, [and] natural language processing to drive more efficiency and insights into the process as well as AI and ML [machine learning] to deliver predictive analytics, alerting, and automated notifications about outliers or data discrepancies. For example, from natural language processing they’re looking at, How can I use natural language processing for document review, ingestion, and analysis, when I have to bring tax notices into my system? Or, How can I use automation with predefined, process-specific workflows to make it easier for the teams involved to stay in compliance with the tax filings and related deadlines? At a macro level, I think, Michael, what has really changed is the need for continuous efficiency improvements, combined with the breadth, the scope of the data, and the complexity that can be introduced by changing regulations.
Ramping Up Your Digital Transformation Game
Levin-Epstein: You’ve clearly identified the why. I think our members would be interested in knowing what they should be doing to ramp up to meet this digital transformation. Is there an area where you think they could be doing better?
Singh: Absolutely. I’ve done this previously—digital transformation across travel—which along with retail has undergone a significant digital transformation. If you look at travel, for example, online travel agencies, such as Travelocity and Expedia, emerged on the scene maybe—I may be slightly off by a few years here—about twenty years ago. Earlier, we used to call [travel] agents, and the agent was the only one who had the terminal that connected with Sabre’s or Amadeus’ global distribution ticketing system, depending on where you lived in the world. And the agent would book that ticket for you. Same thing for hotels. And now, if you look at it, we can go to sites like Expedia, Travelocity, and Kayak ourselves and make the entire booking ourselves, including selecting our seats and even the food we want to eat. The extent of digital transformation these companies went through is such that they can offer price differences based on various characteristics of the shopper that arrives on their site. A big part of this transformation has been how these companies managed their data—across visitors, customers, and suppliers.
To that extent, we can draw some learning and parallels here for organizations from a digitization of tax and trade operations. First and foremost, for digitization, organizations need to have a clear view of their data sources and data flow. The questions that can help here are, How is my data structured? Where is my data housed? Do I have a clear strategy to integrate, clean, and store the data, [and] grant access to it in a compliant manner, so that I can create the highest order of value from it appropriately? That is first and foremost. Once organizations have a clear data strategy, that’s the foundational layer, if you think about it as a stack. This bottommost layer is where the data integration, management, and storage reside. Second layer on top of that is business intelligence and reporting. What packaged, out-of-the-box, standardized insights am I providing on the data? Are we making it easier for people to self-service to get custom insights on the data to transform into business advisors? Then, the third layer of the stack is where we get into things like AI and ML and move into predictive, forward-looking application of the data. How do I leverage data that has typically been used from a descriptive, looking-in-the-past perspective and start building use cases on top of it that are forward-looking and predictive for planning purposes? To expand beyond tax filing into tax planning and advisory, if you will. Then you draw a circle around this three-layer stack that represents technologies such as robotic process automation, APIs, and collaboration. APIs enable the organization to integrate insights, predictions, and alerts into applications to leverage during key workflows, and RPA [robotic process automation] delivers automation of the workflows from an efficiency and accuracy perspective. Collaboration extends the benefit of these services across various parties of the value chains—whether it is finance and accounting teams working together for tax workflows or global trade and indirect tax teams working on total landed cost.
ML, AI, and Robotic Processing
Levin-Epstein: Not everyone really understands ML and AI and robotic processing, and I think it would be very helpful if you could explain those concepts a little bit more.
Singh: Sure, absolutely. Let’s take the example of indirect tax, like sales tax or VAT tax. If you look at the Wayfair ruling, which basically created the concept of economic nexus, of sales thresholds beyond which a corporate entity has a legal obligation to collect and remit sales taxes. Any company that has e-commerce operations basically is impacted by the ruling around economic nexus. Given the compliance implications of this ruling, as a company, how do you ensure that you have insights into your sales and understand when you might cross the threshold? First, you need to make sure that your sales and sales tax data is coming into that one central layer, the bottom data layer that I described earlier, to make sure that you have your full visibility of sales that you’re making in any given state. Because if you don’t have a clear view of all the sales you’re making in a given state, your read [on] the related sales tax implications may be incorrect. You may assume that you’re not crossing the economic nexus threshold, but in reality you may have because you didn’t have the right data sets coming in one single place. That’s one. Second is that now, once you have the data coming in one central place, this is where you can build a system that automatically analyzes the current sales data, extrapolates it to predict future sales trends, and compares it against the regional nexus requirements to determine when the organization is going to cross the threshold based on existing sales trends. So, it alerts the organization, in a predictive manner, that, on such-and-such date or in such-and-such time, you are going to cross that threshold. That’s the power of predictive analytics, that it can help scale the analysis across localities and regions and alerts the organization in a proactive manner to avoid complications and being on the wrong side of compliance. That’s one example on the indirect tax side. There are similar examples on the global trade side, and I am happy to talk about it if there’s interest and benefit for your readers.
Global Trade
Levin-Epstein: If you could do the same thing you just did on indirect tax on the global trade side, I think that would be very helpful.
Singh: On the global trade side, the pandemic and the current situation in Ukraine have brought to the front, for companies, the need for supply chain resiliency. This means that companies need to understand the potential risks in their supply chain and plan around them to avoid disruption from supplier-specific risks, geopolitical destabilization, adverse weather events, or other factors that may impact the availability of the materials required by their suppliers for delivery of goods. Companies that have cross-border movement of goods or source components, parts, internationally need to have visibility into the various elements of risks into their supply chain. To expand on one of the aspects I talked about earlier, most companies typically will have more than one tier of suppliers. This first tier of suppliers—the companies they are most engaged with and have contractual agreements with directly—and therefore it is not quite often where unknown risks lie. The risks increase when you go into other tiers, the third, the fourth, the fifth—the further away you get from the first tier, the higher the potential lack of visibility into the supplier and their operations, and the higher the risk. Companies need visibility into these suppliers: Are these good or bad actors that we are dealing with, are they potentially on some form of denied party list by the governments, are they sourcing their materials ethically and legally, are they following the rules when it comes to their labor force, are they financially solvent to continue to fulfill my orders, are there any risks with where they are located physically due to geopolitical tensions or weather-related issues? This is an exercise in acquiring, assimilating, and leveraging data from multiple different sources; it is an important ingredient in ensuring that the supply chain keeps humming smoothly even when faced with temporary fluctuations. Given the variety and volume of the related data, companies often need to utilize external services to gather and bring together such data for them to integrate into their supply chain and global trade applications. And this can take the form of either APIs that are integrated by the company into their applications or a software purchase that offers such data and integrations into their software application for global trade and supply chain management.
Levin-Epstein: The final question has to do with putting together the team to deal with digital transformation—who should be on this team?
Singh: The first and foremost task for any digital transformation should be to evaluate the existing workflow and needs of your “internal customers.” To draw a parallel, as a product leader, it should be all about the needs of your customer. In this case, the customer, the internal functional teams for whom digital transformation will create the biggest positive impact—whether that be the tax team, sourcing team, procurement team or the [financial planning and analysis] team who are evaluating total landed cost implications—they are the first and foremost team members who should be part of this cross-functional team, call it a “squad,” focused on digital transformation. The second team is the digital team that is reviewing the existing construct of workflow and processes and maps out the opportunities to substantially transform, improve upon it, by leveraging digital applications and infrastructure and mapping digital processes. In addition, the digital team needs to determine the “build/buy” path for software applications. Since we are talking about applications and infrastructure, we need to ensure that there is representation from the technology team in this squad. The technology team is focused on data management, analytics/AI, application integrations, information sharing, and collaboration both internally and externally. The final team I’d add to the squad are the data scientists. They are the ones who generate value for transformation initiatives by shifting the focus from descriptive into predictive insights. This is the team that looks at the data that has been collected and works with the functional teams to define predictive insights and opportunities for process efficiency. To summarize, the squad for digital transformation should include the functional stakeholder as the customer, digital team, technology team, and the data science team.
Levin-Epstein: Thank you.